AI in Cognitive Neuroscience: Mapping the Brain's Functions
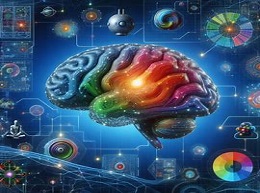
The human brain, with its intricate networks of neurons and synapses, remains one of the most complex and enigmatic structures in the known universe. Unlocking its mysteries has been a longstanding goal of cognitive neuroscience, and artificial intelligence (AI) is proving to be an invaluable tool in this endeavor. By leveraging AI algorithms and advanced imaging techniques, researchers are gaining unprecedented insights into the brain's functions, paving the way for groundbreaking discoveries in neuroscience and cognitive science. In this article, we delve into the intersection of AI and cognitive neuroscience, exploring how AI is revolutionizing our understanding of the brain's inner workings.
Mapping Brain Structure with AI
One of the primary applications of AI in cognitive neuroscience is in mapping the structure of the brain. Advanced imaging technologies, such as functional magnetic resonance imaging (fMRI) and diffusion tensor imaging (DTI), generate vast amounts of complex data about the brain's anatomy and connectivity. AI algorithms can analyze this data to identify patterns, relationships, and abnormalities in brain structure.
Example: Connectomics
Connectomics is a field of neuroscience that aims to map the brain's connectome—the complete set of neural connections or "wiring diagram" of the brain. AI algorithms play a crucial role in analyzing the massive datasets generated by connectome imaging techniques, such as diffusion MRI, to reconstruct neural pathways and networks with unprecedented detail and accuracy. This enables researchers to study how information is processed and transmitted across different regions of the brain, shedding light on cognitive functions such as perception, memory, and decision-making.
Understanding Brain Function with AI
In addition to mapping brain structure, AI is also helping researchers unravel the complex dynamics of brain function. By analyzing patterns of neural activity recorded from electroencephalography (EEG) or magnetoencephalography (MEG) data, AI algorithms can decipher the neural signatures underlying various cognitive processes and behaviors.
Example: Decoding Thoughts
Researchers have used machine learning algorithms to decode brain activity patterns associated with specific thoughts, perceptions, or intentions. For example, studies have shown that AI can reconstruct visual images or spoken words from brain activity patterns recorded using fMRI or EEG, offering insights into the neural basis of perception and language processing. These advances have implications for developing brain-computer interfaces (BCIs) that enable direct communication between the brain and external devices, opening up new possibilities for assistive technologies and neuroprosthetics.
Predicting and Diagnosing Brain Disorders
AI is also being used to predict and diagnose brain disorders such as Alzheimer's disease, Parkinson's disease, and schizophrenia. By analyzing multimodal brain imaging data and clinical variables, AI algorithms can identify biomarkers and patterns associated with different neurological and psychiatric conditions, enabling early detection and personalized treatment strategies.
Example: Predicting Alzheimer's Disease
Researchers have developed AI models that can predict the onset and progression of Alzheimer's disease based on brain imaging data and cognitive assessments. These models analyze patterns of brain atrophy, metabolic changes, and cognitive decline to identify individuals at high risk of developing Alzheimer's disease years before symptoms manifest. Early intervention based on these predictions can potentially delay or mitigate the progression of the disease, improving outcomes for patients and their families.
Challenges and Ethical Considerations
While AI holds great promise for advancing cognitive neuroscience, it also raises important challenges and ethical considerations. One concern is the interpretability of AI models in neuroscience—how do we understand and trust the insights generated by AI algorithms about the brain's functions and dysfunctions?
Interpretable AI in Neuroscience
Researchers are developing methods to enhance the interpretability of AI models in neuroscience, such as visualization techniques that highlight relevant features or connections in brain imaging data. By making AI-generated insights more transparent and understandable, researchers can validate findings, generate new hypotheses, and guide future experiments in cognitive neuroscience.
Ethical Use of Brain Data
Another challenge is ensuring the ethical use of brain data in AI research. As the collection and analysis of brain imaging data become more widespread, it is essential to protect the privacy and confidentiality of research participants, adhere to informed consent principles, and establish guidelines for responsible data sharing and usage in neuroscience research.
Artificial intelligence is revolutionizing cognitive neuroscience, empowering researchers to unlock the secrets of the human brain with unprecedented precision and insight. By leveraging AI algorithms and advanced imaging techniques, scientists are mapping the brain's structure, deciphering its functions, and predicting and diagnosing brain disorders with greater accuracy and efficiency than ever before. However, it is essential to address the challenges and ethical considerations associated with AI in neuroscience, ensuring transparency, accountability, and responsible use of brain data. By harnessing the power of AI responsibly and ethically, we can continue to unravel the mysteries of the brain and advance our understanding of cognition, behavior, and consciousness.