AI in Geology: Applications in Earth Sciences and Exploration
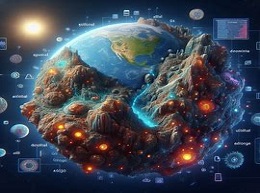
Artificial intelligence (AI) is transforming the field of geology, offering innovative solutions to complex challenges in earth sciences and exploration. From predicting natural disasters to identifying mineral deposits, AI technologies enhance the accuracy, efficiency, and scope of geological investigations. In this article, we explore the diverse applications of AI in geology and its implications for the future of earth sciences.
Seismic Analysis and Earthquake Prediction
AI algorithms analyze seismic data to detect patterns and precursors of earthquakes, enabling scientists to predict and mitigate the impact of seismic events. Machine learning models trained on historical earthquake data can identify seismic signatures and predict the likelihood of future earthquakes in specific regions. By providing early warning systems and informing disaster preparedness efforts, AI-driven seismic analysis contributes to public safety and resilience in earthquake-prone areas.
Example: The California Integrated Seismic Network (CISN) utilizes AI algorithms to analyze real-time seismic data from a network of sensors across the state. By detecting subtle changes in seismic activity, CISN can issue timely alerts and notifications to residents and emergency responders, helping mitigate the impact of earthquakes and reduce the risk of casualties and damage.
Remote Sensing and Geological Mapping
AI technologies enhance remote sensing techniques for geological mapping and exploration. Satellite imagery and aerial surveys are processed using machine learning algorithms to identify geological features, mineral deposits, and environmental changes. AI-driven image analysis tools can distinguish subtle spectral signatures and geological patterns that may indicate the presence of valuable resources or geological hazards.
Example: Rio Tinto, a mining company, employs AI-powered remote sensing technologies to explore and map mineral deposits in remote and inaccessible regions. By analyzing satellite imagery and geospatial data, Rio Tinto can identify promising exploration targets and optimize resource extraction strategies, reducing costs and environmental impact.
Reservoir Characterization and Oil Exploration
AI facilitates reservoir characterization and oil exploration by analyzing geological data and optimizing drilling operations. Machine learning algorithms analyze seismic data, well logs, and geological surveys to model subsurface reservoirs and predict hydrocarbon reservoir properties. AI-driven reservoir characterization tools enable oil and gas companies to identify prospective drilling locations and optimize production strategies, maximizing resource recovery and profitability.
Example: ExxonMobil utilizes AI-powered reservoir characterization techniques to optimize oil and gas exploration and production operations. By integrating seismic data, well logs, and geological models, ExxonMobil can identify promising drilling locations, mitigate drilling risks, and enhance reservoir performance, ensuring efficient and sustainable resource extraction.
Geological Hazard Assessment and Risk Management
AI technologies support geological hazard assessment and risk management by analyzing geological data and modeling potential hazards. Machine learning algorithms can predict landslides, volcanic eruptions, and other geological hazards by analyzing historical data and environmental factors. AI-driven risk assessment tools enable policymakers, urban planners, and emergency responders to develop mitigation strategies and improve resilience to geological hazards.
Example: The Geological Survey of Japan utilizes AI algorithms to assess landslide risks and develop hazard maps for vulnerable areas. By analyzing topographic data, rainfall patterns, and historical landslide events, the Geological Survey of Japan can identify areas at high risk of landslides and implement preventive measures to protect lives and property.
Despite its transformative potential, the integration of AI in geology presents certain challenges and considerations. Data quality, accessibility, and interpretation remain key obstacles to AI-driven geological analyses, requiring collaboration between geologists, data scientists, and technology developers to address. Additionally, ethical considerations regarding data privacy, bias, and transparency must be addressed to ensure responsible AI deployment in geological applications.
As AI technologies continue to advance, the future of geology holds promise for innovative solutions to pressing environmental and resource challenges. By leveraging AI to enhance geological analyses, exploration techniques, and hazard assessments, scientists can deepen our understanding of the Earth and contribute to sustainable development and environmental stewardship.