AI in Pharmaceuticals: Drug Discovery and Development
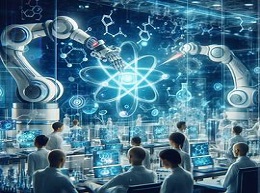
AI in Pharmaceuticals: Revolutionizing Drug Discovery and Development
Artificial intelligence (AI) has emerged as a game-changer in the pharmaceutical industry, transforming traditional drug discovery and development processes. This article provides an in-depth exploration of the role of AI in pharmaceuticals, highlighting its applications, benefits, and real-world examples.
AI in Pharmaceuticals: Pharmaceutical research and development (R&D) is a complex and costly endeavor, with drug discovery and development processes typically taking years and requiring significant financial investments. AI technologies, such as machine learning, deep learning, and natural language processing, offer innovative solutions to streamline these processes, accelerate drug discovery, and improve therapeutic outcomes.
AI Applications in Drug Discovery:
- Virtual Screening: AI algorithms analyze large databases of chemical compounds to predict their potential efficacy as drug candidates, significantly reducing the time and resources required for traditional laboratory-based screening.
- Target Identification and Validation: AI models identify disease targets and validate their relevance in the context of specific diseases, facilitating the selection of promising drug targets for further investigation.
- De Novo Drug Design: AI-driven algorithms generate novel chemical structures with desired pharmacological properties, enabling the rapid design of innovative drug candidates tailored to specific therapeutic targets.
- Drug Repurposing: AI analyzes existing drugs and their molecular mechanisms to identify new therapeutic applications, accelerating the repurposing of approved drugs for the treatment of different diseases.
AI in Clinical Trials and Drug Development:
- Patient Recruitment: AI platforms leverage clinical and genomic data to identify eligible patients for clinical trials more efficiently, speeding up the recruitment process and ensuring the inclusion of diverse patient populations.
- Predictive Analytics: AI algorithms analyze patient data to predict treatment outcomes, adverse drug reactions, and disease progression, helping pharmaceutical companies optimize clinical trial design and make data-driven decisions.
- Personalized Medicine: AI enables the development of personalized treatment regimens based on individual patient characteristics, such as genetic makeup, biomarker profiles, and lifestyle factors, improving treatment efficacy and patient outcomes.
Benefits of AI in Pharmaceuticals:
- Accelerated Drug Discovery: AI-powered platforms can sift through vast amounts of data and identify promising drug candidates in a fraction of the time it takes using traditional methods.
- Cost Savings: By reducing the time and resources required for drug discovery and development, AI can lower R&D costs and potentially decrease the overall cost of bringing new drugs to market.
- Enhanced Precision and Efficacy: AI-driven drug discovery approaches enable the development of more targeted therapies with improved efficacy and reduced side effects, leading to better patient outcomes.
Real-World Examples of AI in Pharmaceuticals:
- Atomwise: Atomwise's AI platform uses deep learning models to predict the binding affinity of small molecules to protein targets, facilitating drug discovery for various diseases, including cancer, Alzheimer's, and infectious diseases.
- BenevolentAI: BenevolentAI's AI platform analyzes biomedical data to identify novel drug targets and repurpose existing drugs for new indications, accelerating the development of treatments for conditions such as amyotrophic lateral sclerosis (ALS) and Parkinson's disease.
- Recursion Pharmaceuticals: Recursion Pharmaceuticals utilizes AI-powered image analysis and machine learning algorithms to screen thousands of compounds for potential drug candidates, focusing on rare genetic diseases and oncology.
Challenges and Future Directions:
- Data Quality and Accessibility: Access to high-quality, standardized data remains a challenge in AI-driven drug discovery, requiring collaboration between pharmaceutical companies, academic institutions, and regulatory agencies to address data sharing and interoperability issues.
- Regulatory Considerations: As AI-driven drug discovery methods gain prominence, regulatory agencies face the challenge of adapting existing regulations to accommodate these innovative approaches while ensuring patient safety and efficacy.
- Ethical and Legal Implications: The use of AI in pharmaceuticals raises ethical concerns related to data privacy, algorithm bias, and the responsible use of AI-driven insights, necessitating the development of ethical guidelines and regulatory frameworks to govern AI applications in healthcare.
In conclusion, AI is revolutionizing the pharmaceutical industry by accelerating drug discovery, improving clinical trial efficiency, and enabling the development of personalized therapies. Despite challenges and ethical considerations, the integration of AI into pharmaceutical R&D holds immense promise for addressing unmet medical needs and improving global public health.