AI in Quantum Chemistry: Accelerating Drug Discovery and Materials Science
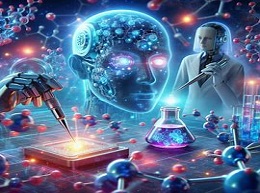
AI in Quantum Chemistry: Revolutionizing Discovery
In the realm of quantum chemistry, where the complexities of molecular interactions often defy traditional computation methods, Artificial Intelligence (AI) emerges as a game-changer. By harnessing the power of AI, researchers can accelerate drug discovery and materials science, paving the way for groundbreaking innovations. In this article, we delve into the synergy between AI and quantum chemistry, exploring its transformative impact on scientific discovery and technological advancement.
Quantum Chemistry: Quantum chemistry is a branch of chemistry that applies quantum mechanics principles to understand and predict the behavior of molecules and chemical reactions. At the molecular level, the behavior of atoms and electrons is governed by quantum mechanics, which describes the probabilistic nature of particles and their interactions. Understanding molecular structures, properties, and dynamics is crucial for various fields, including drug discovery, materials science, and environmental research. However, the computational complexity of quantum systems presents significant challenges for traditional simulation methods.
Challenges in Quantum Chemistry: Quantum chemistry calculations involve solving the Schrödinger equation, which describes the quantum state of a system, to determine molecular properties such as energy, structure, and reactivity. However, the computational cost of solving the Schrödinger equation scales exponentially with the number of atoms and electrons in a molecule, making accurate predictions for large systems impractical with classical computers. As a result, researchers often resort to approximations and simplifications that limit the accuracy and applicability of their models.
AI-Powered Quantum Chemistry: AI offers a promising approach to overcome the limitations of traditional quantum chemistry methods by leveraging machine learning algorithms to accelerate computations and improve accuracy. Machine learning models trained on large datasets of quantum chemical calculations can learn to predict molecular properties with high accuracy and efficiency, bypassing the need for costly quantum simulations. For example, neural networks and Gaussian process regression models can predict molecular energies, geometries, and spectra with comparable accuracy to state-of-the-art quantum chemistry methods but at a fraction of the computational cost.
Accelerating Drug Discovery: In drug discovery, AI-driven quantum chemistry accelerates the screening of candidate compounds and the optimization of molecular structures for desired properties. By predicting the binding affinity of drug molecules to target proteins, AI models help identify promising drug candidates with the potential to treat diseases more effectively and with fewer side effects. Moreover, AI algorithms can explore vast chemical space and suggest novel drug candidates with desired pharmacological properties, facilitating the discovery of new therapeutic agents for challenging diseases.
Materials Design and Discovery: In materials science, AI-enabled quantum chemistry accelerates the design and discovery of new materials with tailored properties for various applications, including energy storage, catalysis, and electronics. Machine learning models trained on quantum mechanical data can predict material properties such as bandgap, conductivity, and stability, guiding the search for materials with optimal performance and efficiency. Furthermore, AI-driven materials discovery platforms enable researchers to explore novel material compositions and structures that exhibit unprecedented properties, revolutionizing the development of advanced materials for emerging technologies.
Future Outlook and Challenges: The synergy between AI and quantum chemistry holds immense potential to revolutionize scientific discovery and technological innovation in the coming years. However, significant challenges remain, including the need for large, high-quality datasets, robust machine learning algorithms, and interpretable models. Additionally, integrating AI-driven quantum chemistry into existing research workflows and ensuring reproducibility and reliability are essential for realizing its full potential in drug discovery, materials science, and beyond.
In conclusion, AI-powered quantum chemistry represents a paradigm shift in scientific research, offering unprecedented capabilities to accelerate drug discovery, materials science, and other fields. By combining the predictive power of machine learning with the accuracy of quantum mechanics, researchers can unlock new possibilities for innovation and address pressing challenges in healthcare, energy, and environmental sustainability. As AI continues to advance, its impact on quantum chemistry promises to reshape our understanding of molecular phenomena and drive the development of transformative technologies for the benefit of society.