AI in Radiology: Transforming Medical Imaging and Diagnostics
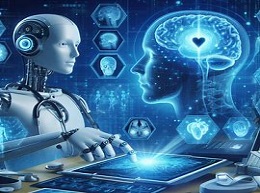
The Role of AI in Radiology
Artificial Intelligence (AI) is rapidly transforming the field of radiology, revolutionizing medical imaging and diagnostics. With the ability to analyze vast amounts of data and identify patterns that may elude the human eye, AI technologies are enhancing the accuracy and efficiency of radiological interpretation, leading to improved patient outcomes. In this article, we delve into the impact of AI on radiology, exploring its applications, benefits, and potential challenges.
Advancements in Medical Imaging
Medical imaging plays a crucial role in the diagnosis and treatment of various medical conditions, ranging from fractures and tumors to cardiovascular diseases and neurological disorders. Traditional imaging techniques, such as X-rays, CT scans, MRI, and ultrasound, provide valuable insights into the internal structures of the body. However, interpreting these images accurately can be challenging, requiring specialized training and expertise.
AI-Powered Image Analysis
AI algorithms can analyze medical images with remarkable speed and accuracy, assisting radiologists in detecting abnormalities, diagnosing diseases, and planning treatment strategies. Deep learning algorithms, in particular, excel at pattern recognition tasks, allowing them to identify subtle signs of disease that may be overlooked by human observers. By leveraging AI-powered image analysis, radiologists can expedite the interpretation process and provide more timely and accurate diagnoses.
Applications of AI in Radiology
AI has numerous applications in radiology, spanning a wide range of imaging modalities and clinical scenarios. Some common applications include:
-
Detection of Abnormalities: AI algorithms can detect abnormalities in medical images, such as tumors, fractures, and anomalies, with high sensitivity and specificity.
-
Quantitative Analysis: AI enables quantitative analysis of imaging biomarkers, providing objective measurements of disease severity and progression.
-
Image Reconstruction: AI techniques can enhance image quality and reduce noise in medical images, improving visualization and diagnostic accuracy.
-
Computer-Aided Diagnosis: AI-powered computer-aided diagnosis (CAD) systems assist radiologists in interpreting medical images by highlighting suspicious findings and providing diagnostic support.
Examples of AI in Radiology
Example 1: DeepMind's AI for Retinal Scans
DeepMind, a subsidiary of Alphabet Inc., developed an AI system capable of analyzing retinal scans to detect early signs of eye diseases such as diabetic retinopathy and macular degeneration. The AI system achieved performance comparable to expert ophthalmologists, demonstrating the potential for AI to improve the early detection and management of eye diseases.
Example 2: Aidoc's AI for CT Scans
Aidoc, an AI-powered medical imaging company, developed algorithms to analyze CT scans and identify abnormalities such as intracranial hemorrhage, pulmonary embolism, and fractures. By flagging critical findings for radiologists' attention, Aidoc's AI system helps expedite diagnosis and treatment, particularly in emergency settings where timely intervention is crucial.
Challenges and Considerations
While AI holds great promise for enhancing radiological practice, it also presents challenges and considerations that must be addressed:
-
Data Quality and Bias: AI algorithms rely on high-quality, diverse datasets for training. Ensuring the representativeness and fairness of training data is essential to mitigate biases and improve algorithm performance.
-
Regulatory Approval: AI-based medical devices and algorithms must undergo rigorous validation and regulatory approval processes to ensure their safety, efficacy, and compliance with healthcare regulations.
-
Integration with Clinical Workflow: Integrating AI tools seamlessly into the radiology workflow requires careful consideration of user interface design, workflow integration, and interoperability with existing systems.
Future Directions: Towards Precision Radiology
As AI continues to evolve, the future of radiology holds promise for even more advanced imaging techniques and personalized treatment strategies. By harnessing the power of AI to analyze medical images and extract actionable insights, radiologists can deliver more precise diagnoses, tailor treatment plans to individual patient needs, and improve overall healthcare outcomes.
Harnessing the Power of AI in Radiology
AI is revolutionizing the field of radiology, empowering radiologists with advanced tools and techniques for image interpretation and diagnosis. By augmenting human expertise with AI-powered image analysis, radiologists can enhance diagnostic accuracy, expedite treatment decisions, and improve patient care. As AI technologies continue to mature, the future of radiology holds exciting possibilities for transforming medical imaging and diagnostics, ultimately leading to better healthcare outcomes for patients worldwide.