Discussing Bias in AI: Challenges and Mitigation Strategies
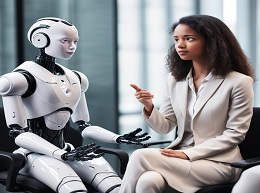
Artificial Intelligence (AI) has the potential to revolutionize various aspects of society, from healthcare to finance. However, the deployment of AI systems also comes with inherent risks, particularly the risk of bias. In this article, we delve into the complexities of bias in AI, its impact on society, and strategies to mitigate bias for more fair and equitable AI systems.
Understanding Bias in AI
Bias in AI refers to systematic and unfair favoritism or discrimination towards certain groups or individuals in AI systems' decision-making processes. This bias can manifest in various forms, including racial bias, gender bias, socioeconomic bias, and cultural bias. It stems from the inherent limitations of AI algorithms, as well as the data used to train and test these algorithms.
Example: Racial Bias in Facial Recognition
Facial recognition systems have been shown to exhibit racial bias, with higher error rates for individuals with darker skin tones. This bias can lead to discriminatory outcomes, such as misidentification and wrongful arrests, disproportionately affecting marginalized communities.
Impact of Bias in AI
Bias in AI can have far-reaching consequences across various domains, including employment, criminal justice, healthcare, and finance. Biased AI systems can perpetuate and exacerbate existing inequalities and discrimination, leading to unfair treatment, lack of access to opportunities, and erosion of trust in AI technologies.
Example: Bias in Hiring Algorithms
AI-powered hiring algorithms may inadvertently perpetuate gender or racial bias in recruitment processes by favoring candidates from certain demographics or penalizing candidates with non-traditional backgrounds. This bias can result in unequal employment opportunities and reinforce existing disparities in the workforce.
Challenges in Addressing Bias
Addressing bias in AI poses several challenges, including the complexity of AI algorithms, the opacity of decision-making processes, and the lack of diverse and representative datasets. Additionally, biases embedded in societal structures and historical data can inadvertently perpetuate bias in AI systems, making it difficult to identify and mitigate bias effectively.
Example: Algorithmic Transparency
Many AI algorithms, particularly deep learning models, operate as black boxes, making it challenging to interpret their decision-making processes and identify sources of bias. Lack of transparency in AI systems hinders efforts to detect and address bias effectively, leading to potential unintended consequences.
Mitigation Strategies for Bias in AI
Despite the challenges, various strategies can help mitigate bias in AI systems and promote fairness and equity. These strategies include diverse and representative dataset collection, algorithmic fairness techniques, bias detection and mitigation tools, and interdisciplinary collaboration between AI researchers, ethicists, policymakers, and community stakeholders.
Example: Fairness-Aware Machine Learning
Fairness-aware machine learning techniques aim to mitigate bias in AI algorithms by explicitly considering fairness constraints during model training and evaluation. These techniques ensure that AI systems make decisions that are equitable and unbiased across different demographic groups, mitigating the risk of discriminatory outcomes.
Collaborative Efforts for Ethical AI
Addressing bias in AI requires collaborative efforts from various stakeholders, including AI developers, policymakers, regulators, and community advocates. By fostering interdisciplinary collaboration and adopting ethical guidelines and standards, we can ensure that AI technologies are developed and deployed in a responsible and equitable manner, promoting societal well-being and inclusivity.
Example: Partnership for AI Ethics
The Partnership for AI Ethics is a collaborative initiative that brings together industry leaders, researchers, and civil society organizations to develop ethical guidelines and best practices for responsible AI development and deployment. By fostering dialogue and collaboration, the partnership aims to promote ethical AI practices and mitigate the risks of bias and discrimination in AI systems.
Conclusion: Towards Ethical and Fair AI
Bias in AI is a complex and multifaceted issue that requires careful consideration and proactive measures to address effectively. By understanding the challenges of bias in AI, implementing mitigation strategies, and fostering collaborative efforts, we can ensure that AI technologies promote fairness, equity, and inclusivity, advancing the promise of AI for the betterment of society.
In conclusion, discussing bias in AI is crucial for promoting ethical and fair AI systems that uphold human values and respect diversity. By addressing bias in AI proactively and collaboratively, we can harness the transformative potential of AI to create a more just, equitable, and inclusive future for all.