Explaining AI: Demystifying Complex Models
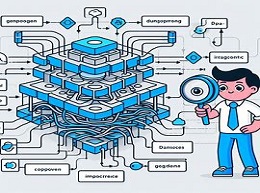
Discover the importance of Explainable AI (XAI) in making opaque machine learning models transparent and understandable to users and stakeholders.
1. Understanding Explainable AI Explore the concept of Explainable AI (XAI) and its significance in interpreting how AI models make decisions. Learn about the need for transparency and accountability in AI systems.
2. Techniques for Model Interpretability Discover various techniques used in Explainable AI (XAI) to enhance model interpretability, including feature importance analysis, model visualization, and surrogate models.
3. Local vs. Global Interpretability Differentiate between local and global interpretability in AI models. Understand how local interpretability focuses on explaining individual predictions, while global interpretability provides insights into the overall model behavior.
4. Interpretable Machine Learning Algorithms Explore machine learning algorithms known for their interpretability, such as decision trees, linear models, and rule-based systems. Learn how these algorithms facilitate understanding and trust in AI systems.
5. Post-hoc Explainability Techniques Discover post-hoc explainability techniques used to explain the decisions of complex black-box models, including LIME (Local Interpretable Model-Agnostic Explanations) and SHAP (SHapley Additive exPlanations).
6. Real-World Applications of Explainable AI Explore examples of how Explainable AI (XAI) is applied in various domains, such as healthcare, finance, and autonomous vehicles. Learn how transparent AI systems enhance trust and usability.
7. Challenges and Limitations Discuss the challenges and limitations of Explainable AI (XAI), including trade-offs between interpretability and model performance, as well as the potential impact on model complexity.
8. Ethical Considerations Examine the ethical considerations surrounding Explainable AI (XAI), including privacy concerns, algorithmic bias, and the right to explanation. Explore strategies for mitigating these ethical challenges.
9. Future Directions Discuss future directions in Explainable AI (XAI), including advancements in interpretability techniques, regulatory frameworks, and the integration of human-centric design principles.
In conclusion, Explainable AI (XAI) plays a crucial role in fostering trust, understanding, and accountability in AI systems. By prioritizing transparency and interpretability, we can empower users to confidently leverage the benefits of AI while mitigating risks and ensuring ethical AI adoption.