Exploring AI in Materials Science: Innovations in Material Discovery
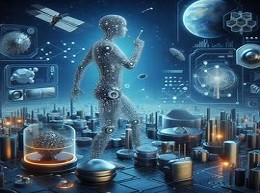
Materials science is a field at the forefront of innovation, driving advancements in various industries, from electronics to healthcare. The discovery of new materials with desirable properties is crucial for developing next-generation technologies. In recent years, artificial intelligence (AI) has emerged as a powerful tool in materials science, revolutionizing the process of material discovery. In this article, we explore the role of AI in accelerating innovation and unlocking new possibilities in materials science.
AI in Materials Design and Discovery
Traditional methods of materials discovery rely on trial and error experiments, which can be time-consuming and costly. AI offers a more efficient approach by leveraging machine learning algorithms to predict the properties of materials based on their chemical composition and structure. By analyzing vast databases of materials data, AI algorithms can identify patterns and correlations that lead to the discovery of novel materials with tailored properties.
Example: Researchers at Stanford University developed a machine learning model called Atom2Vec, which learns the relationships between atoms in a material's structure and its properties. By analyzing the atomic structure of known materials, Atom2Vec can predict the properties of hypothetical materials with high accuracy, expediting the discovery of new materials for applications such as batteries and catalysts.
High-Throughput Screening and Virtual Experiments
AI enables high-throughput screening of materials by simulating virtual experiments and predicting their properties computationally. Quantum mechanical simulations, molecular dynamics, and density functional theory calculations are combined with machine learning algorithms to evaluate the performance of materials under various conditions. This approach accelerates the discovery process by prioritizing promising candidates for experimental validation.
Example: The Materials Project, a collaborative effort led by Lawrence Berkeley National Laboratory, utilizes AI-driven computational tools to screen millions of materials for various applications. By simulating the properties of materials in silico, researchers can identify promising candidates for energy storage, electronic devices, and structural materials, significantly reducing the time and cost of experimental research.
Predictive Modeling and Property Optimization
AI enables predictive modeling of material properties, allowing researchers to design materials with specific characteristics for targeted applications. Machine learning algorithms analyze the relationships between material composition, processing parameters, and performance metrics to optimize material properties. This approach facilitates the development of materials with enhanced strength, conductivity, and durability for diverse applications.
Example: Airbus employs AI-driven predictive modeling tools to optimize the performance of aerospace materials. By analyzing data from material tests, simulations, and manufacturing processes, Airbus can predict the behavior of materials under different operating conditions and design components with improved reliability and efficiency.
Drug Discovery and Materials Informatics
AI-driven materials informatics platforms integrate data from diverse sources, including experimental measurements, scientific literature, and materials databases, to facilitate data-driven decision-making in materials science. Machine learning algorithms analyze this wealth of information to uncover hidden patterns, correlations, and design principles that guide the discovery of new materials. This approach accelerates innovation and enables researchers to explore a broader range of materials possibilities.
Example: Citrine Informatics developed a materials informatics platform that utilizes AI to accelerate materials discovery and optimization. By integrating data from experiments, simulations, and literature, Citrine's platform enables researchers to search for materials with specific properties, predict material behavior, and design new materials tailored to their applications.
Challenges and Considerations
Despite its transformative potential, the integration of AI in materials science presents certain challenges and considerations. Data quality and quantity are crucial for training accurate machine learning models, requiring collaboration between researchers and data providers to ensure access to high-quality datasets. Additionally, ethical considerations regarding data privacy, bias, and transparency must be addressed to ensure responsible AI deployment in materials science.
AI is revolutionizing materials science by accelerating innovation, enabling the discovery of novel materials with tailored properties for diverse applications. From materials design and discovery to predictive modeling and property optimization, AI-driven technologies empower researchers to explore new frontiers in materials science. As AI continues to advance, the future of materials discovery holds promise for breakthroughs that will drive progress in technology, healthcare, energy, and beyond.