Exploring AI in Renewable Energy Forecasting
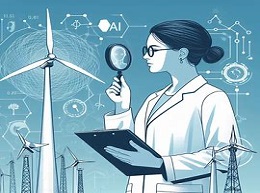
Harnessing AI for Renewable Energy
Renewable energy sources such as solar, wind, and hydroelectric power are increasingly becoming critical components of the global energy landscape. However, the intermittent nature of renewable energy generation poses challenges for grid stability and energy management. In this article, we delve into the role of artificial intelligence (AI) in renewable energy forecasting and its implications for a sustainable energy future.
The Importance of Renewable Energy Forecasting
Accurate forecasting of renewable energy generation is essential for ensuring the reliable and efficient operation of energy systems. By predicting future energy production from sources like solar and wind, grid operators can anticipate fluctuations in supply and demand, optimize energy scheduling, and mitigate potential grid imbalances. Traditionally, renewable energy forecasting relied on statistical models and meteorological data. However, the advent of AI has ushered in a new era of precision forecasting, enabling more reliable and granular predictions.
AI Techniques for Renewable Energy Forecasting
AI offers a diverse range of techniques for renewable energy forecasting, including machine learning algorithms, neural networks, and data-driven models. These techniques leverage historical energy production data, weather forecasts, geographical features, and other relevant factors to generate accurate predictions. For example, recurrent neural networks (RNNs) can analyze time-series data to capture temporal dependencies and patterns in energy generation, while ensemble learning methods can combine multiple models to improve forecasting accuracy.
Solar Energy Forecasting
Solar energy forecasting is a crucial aspect of renewable energy management, given the variability of solar irradiance due to factors such as cloud cover and atmospheric conditions. AI-powered forecasting models for solar energy utilize satellite imagery, weather data, and historical solar radiation measurements to predict solar power output with high precision. By incorporating real-time data and adaptive learning algorithms, these models can continuously refine their predictions and adapt to changing environmental conditions.
Wind Energy Forecasting
Wind energy forecasting presents unique challenges due to the complex dynamics of wind patterns and terrain effects. AI-based approaches for wind energy forecasting leverage advanced algorithms such as support vector machines (SVMs) and deep learning architectures to analyze wind speed, direction, and turbulence data. By integrating information from meteorological sensors, Doppler radar, and wind farm monitoring systems, these models can generate accurate short-term and long-term forecasts for wind power generation.
Hydroelectric Energy Forecasting
Hydroelectric power generation relies on water flow rates, reservoir levels, and weather conditions, making accurate forecasting essential for optimizing energy production and water management. AI techniques such as genetic algorithms and fuzzy logic systems can analyze hydrological data, historical flow patterns, and weather forecasts to predict future water availability and hydropower generation capacity. By considering factors such as precipitation, snowmelt, and river basin dynamics, these models enable efficient scheduling of hydropower operations and reservoir management.
Advancing Renewable Energy with AI
In conclusion, AI holds tremendous potential for advancing renewable energy forecasting and accelerating the transition to a sustainable energy future. By harnessing the power of AI-driven predictive analytics, energy stakeholders can optimize resource allocation, improve grid stability, and maximize the integration of renewable energy sources into the electricity grid. With ongoing research and technological innovation, AI continues to play a pivotal role in driving the transformation of the energy sector towards a cleaner, greener, and more resilient future.