The Challenges of Deploying AI in Real-World Scenarios
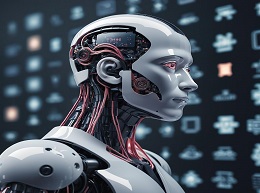
Deploying Artificial Intelligence (AI) in real-world scenarios is a transformative journey fraught with challenges and complexities. This article delves into the practical hurdles encountered when bringing AI into the real world, offering insights through examples that illuminate the intricacies of overcoming these challenges.
1. The Promise and Perils of Real-World AI Deployment:
Embark on the exploration of the challenges that accompany the deployment of AI in the real world. Understand the dichotomy between the promise of AI and the nuanced realities faced in diverse applications.
2. Data Quality and Availability: The Foundation of AI Success:
Delve into the critical role of data in AI deployment. Discuss challenges related to the quality and availability of data, and explore strategies to ensure robust datasets for training and inference.
Example: An AI-driven healthcare system struggling with inconsistent and incomplete medical records, impacting the accuracy of diagnostic predictions.
3. Ethical Considerations and Bias: Navigating the Moral Landscape:
Explore the ethical challenges inherent in AI deployment. Discuss issues of bias, fairness, and transparency, and delve into strategies for addressing these concerns to ensure responsible and equitable AI use.
Example: Facial recognition systems exhibiting bias against certain demographic groups, raising concerns about discriminatory outcomes.
4. Interpretable AI: The Black Box Dilemma:
Dive into the challenge of interpretability in AI models. Discuss the inherent complexity of many AI algorithms and the importance of making their decision-making processes understandable and transparent.
Example: A financial institution struggling to explain AI-driven loan approval decisions, hindering compliance with regulatory requirements.
5. Integration with Existing Systems: The Legacy Conundrum:
Explore the challenges of integrating AI with existing systems and workflows. Discuss issues related to compatibility, scalability, and the need for seamless integration to maximize the impact of AI solutions.
Example: A manufacturing plant facing difficulties integrating AI-powered predictive maintenance into its legacy machinery, leading to operational disruptions.
6. Resource Constraints: The Struggle for Computing Power:
Delve into the resource challenges associated with AI deployment. Discuss the need for significant computing power and explore strategies to navigate resource constraints, especially for small businesses or organizations with limited infrastructure.
Example: A startup developing AI-driven simulations for virtual product testing facing challenges due to limited access to high-performance computing resources.
7. Regulatory Compliance: Navigating the Legal Landscape:
Explore the intricacies of regulatory compliance in AI deployment. Discuss the challenges of adhering to evolving regulations and standards, emphasizing the importance of ethical and legal considerations.
Example: An AI-driven financial advisory service grappling with compliance issues related to data privacy regulations, risking legal repercussions.
8. User Acceptance and Trust: The Human Factor:
Discuss the challenges of gaining user acceptance and trust in AI systems. Explore the importance of human-centric design and transparent communication to foster trust and positive user experiences.
Example: A voice-controlled AI assistant facing resistance due to concerns about privacy invasion, illustrating the need for clear communication about data handling.
9. Continuous Adaptation and Learning: AI in a Dynamic World:
Examine the challenge of ensuring that AI systems adapt to a dynamic and evolving environment. Discuss the need for continuous learning and updates to keep AI models relevant and effective.
Example: An AI-driven chatbot struggling to understand and respond appropriately to newly emerging slang or cultural shifts, highlighting the need for ongoing training.
As AI continues to permeate various sectors, acknowledging and addressing these challenges is essential for successful real-world deployment. Navigating the complexities of data, ethics, integration, resources, regulations, user trust, and continuous adaptation requires a holistic and strategic approach.
"AI Deployment Realities: Navigating Challenges in the Real World" offers a comprehensive exploration of the challenges faced when deploying AI in diverse real-world scenarios. Uncover examples that shed light on the intricacies of overcoming these challenges, paving the way for successful and responsible AI integration.