The Impact of AI on Transportation Infrastructure Planning
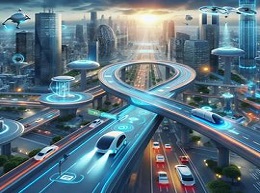
The Impact of AI on Transportation Infrastructure Planning
In the rapidly evolving landscape of transportation, artificial intelligence (AI) is emerging as a transformative force, revolutionizing the way cities plan, design, and manage their transportation infrastructure. This article delves into the profound impact of AI on transportation infrastructure planning, highlighting key advancements, challenges, and real-world examples.
AI in Transportation Planning: Transportation infrastructure planning encompasses the design, construction, and management of roads, bridges, railways, airports, and public transit systems to ensure efficient movement of people and goods. AI technologies, such as machine learning, predictive analytics, and computer vision, are reshaping this field by offering data-driven insights, optimizing routes, and improving decision-making processes.
Optimizing Traffic Flow with AI:
- Traffic Prediction and Management: AI algorithms analyze historical traffic data, weather conditions, and real-time sensor feeds to predict traffic congestion, optimize signal timings, and dynamically adjust traffic flow to minimize delays and improve safety.
- Smart Traffic Lights: AI-powered traffic signal control systems prioritize vehicle and pedestrian movements based on traffic conditions, reducing wait times at intersections and optimizing overall traffic flow.
- Dynamic Route Planning: AI-enabled navigation apps use real-time traffic data and machine learning algorithms to recommend optimal routes, considering factors such as traffic congestion, road closures, and accidents, to minimize travel time and fuel consumption.
- Predictive Maintenance: AI algorithms analyze sensor data from transportation infrastructure assets, such as bridges, tunnels, and railways, to detect anomalies, predict potential failures, and schedule maintenance proactively, ensuring the reliability and safety of critical infrastructure.
Enhancing Public Transit Systems:
- Demand-Responsive Transit: AI-powered algorithms optimize bus routes and schedules based on passenger demand patterns, enhancing the efficiency and accessibility of public transit services, particularly in areas with fluctuating demand.
- Ride-Sharing Services: AI algorithms match passengers with nearby drivers, optimize ride-sharing routes, and dynamically adjust fares based on supply and demand, improving the affordability and availability of ride-sharing services.
- Automated Fare Collection: AI-based fare collection systems streamline the ticketing process, enabling contactless payments, detecting fare evasion, and providing valuable data insights to transit agencies for service planning and optimization.
Urban Planning and Design with AI:
- Traffic Simulation and Modeling: AI-driven simulation tools enable urban planners to assess the impact of new infrastructure projects, such as road expansions or public transit lines, on traffic flow, air quality, and urban mobility patterns, facilitating informed decision-making.
- Pedestrian and Cyclist Safety: AI algorithms analyze traffic camera footage to identify potential safety hazards for pedestrians and cyclists, such as aggressive driving behaviors or inadequate crosswalks, informing urban design interventions to enhance safety.
- Accessibility Planning: AI-powered mapping tools identify barriers to accessibility, such as lack of wheelchair ramps or elevators in public transit stations, and propose infrastructure improvements to ensure equitable access for all members of the community.
Challenges and Ethical Considerations:
- Data Privacy and Security: Collecting and analyzing vast amounts of transportation data raises concerns about privacy infringement and data security breaches, highlighting the importance of robust data protection measures and ethical AI governance frameworks.
- Algorithm Bias: AI algorithms may perpetuate existing biases and inequities in transportation planning, such as favoring affluent neighborhoods over marginalized communities or prioritizing car-centric infrastructure over sustainable modes of transport, necessitating careful consideration of fairness and equity in AI-driven decision-making processes.
Real-World Examples of AI in Transportation Planning:
- Los Angeles Department of Transportation (LADOT): LADOT uses AI-powered traffic signal optimization systems to reduce traffic congestion and improve pedestrian safety at intersections across the city.
- Singapore's Land Transport Authority (LTA): LTA leverages AI-driven predictive maintenance systems to monitor and maintain the city-state's extensive public transit network, ensuring reliable and efficient transportation services for residents and visitors alike.
- Copenhagen's Bicycle Infrastructure Planning: The City of Copenhagen uses AI algorithms to analyze cyclist behavior patterns, identify high-demand cycling routes, and prioritize investments in bicycle infrastructure to promote sustainable urban mobility and reduce traffic congestion.
In conclusion, AI holds tremendous potential to revolutionize transportation infrastructure planning, optimize traffic flow, enhance public transit systems, and shape more sustainable and equitable cities. However, addressing the ethical, privacy, and equity considerations inherent in AI deployment is essential to ensure that AI-driven transportation solutions benefit all members of society.