The Role of AI in Predictive Analytics for Business Decisions
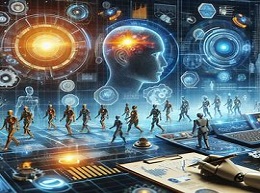
1. The Convergence of AI and Predictive Analytics
Introduce the concept of predictive analytics and highlight the pivotal role of artificial intelligence in enhancing its capabilities for driving business decisions.
2. Understanding Predictive Analytics
Define predictive analytics and discuss its importance in forecasting future outcomes based on historical data patterns, setting the stage for AI integration.
3. AI-Powered Data Processing and Analysis
Explore how AI algorithms process large volumes of data rapidly, identify patterns, and extract insights that traditional analytics methods may overlook.
Example: Highlight how machine learning models analyze customer transaction data to predict future purchasing behavior and optimize marketing strategies.
4. Machine Learning Models for Predictive Modeling
Discuss various machine learning techniques used in predictive modeling, including regression, classification, and clustering algorithms, and their applications in business decision-making.
Example: Showcase how businesses use regression models to forecast sales trends and allocate resources efficiently.
5. Deep Learning for Complex Predictive Tasks
Explain the role of deep learning algorithms, such as neural networks, in handling complex predictive tasks with unstructured data, such as image recognition and natural language processing.
Example: Illustrate how deep learning models analyze medical imaging data to predict patient outcomes and assist physicians in diagnosis and treatment planning.
6. Real-Time Predictive Analytics with AI
Explore how AI enables real-time predictive analytics, allowing businesses to make informed decisions promptly based on up-to-date data streams and dynamic market conditions.
Example: Highlight how financial institutions use AI-powered algorithms to detect fraudulent transactions in real-time and prevent monetary losses.
7. Application Areas of AI-Powered Predictive Analytics
Discuss the diverse application areas of AI-powered predictive analytics across industries, including finance, healthcare, marketing, and manufacturing.
Example: Explore how predictive maintenance systems use AI to anticipate equipment failures in industrial settings, minimizing downtime and maintenance costs.
8. Challenges and Considerations
Address the challenges and considerations associated with implementing AI in predictive analytics, including data quality issues, model interpretability, and ethical concerns.
Example: Discuss the importance of transparency and fairness in AI models to mitigate biases and ensure equitable decision-making.
9. Future Trends and Opportunities
Examine emerging trends and future opportunities in AI-driven predictive analytics, such as the integration of AI with Internet of Things (IoT) devices and the advancement of automated decision-making systems.
Example: Predict the widespread adoption of AI-powered recommendation engines in personalized healthcare and e-commerce platforms.
Conclude by emphasizing the transformative impact of AI in predictive analytics, empowering businesses to make data-driven decisions with confidence and agility.
By exploring the role of AI in predictive analytics, readers gain insights into the potential of artificial intelligence to drive business success and innovation in the digital era.