Understanding Generative Adversarial Networks (GANs) in AI
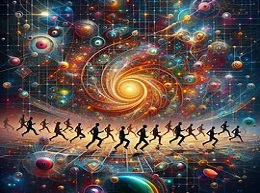
Unveiling the Power of Generative Adversarial Networks (GANs) in AI
Artificial Intelligence (AI) has continuously pushed the boundaries of what's possible, from speech recognition to autonomous vehicles. One of the most intriguing developments within this realm is Generative Adversarial Networks (GANs). This article serves as a comprehensive guide to understanding GANs, exploring their mechanisms, applications, and significance in shaping AI's future.
GANs: Imagine an artist and a critic engaged in a perpetual duel, where the artist constantly strives to create masterpieces while the critic meticulously evaluates each creation. This analogy encapsulates the essence of GANs. Coined by Ian Goodfellow and his colleagues in 2014, GANs consist of two neural networks: the generator and the discriminator.
How GANs Work: The generator's primary role is to produce data, such as images or text, that is indistinguishable from real data. On the other hand, the discriminator's task is to distinguish between real and fake data. Through adversarial training, these networks engage in a constant feedback loop, with the generator refining its output to fool the discriminator, and the discriminator becoming increasingly adept at discerning real from fake.
Applications of GANs: GANs have found applications across various domains, revolutionizing industries and sparking new creative possibilities. One notable application is in image generation, where GANs can create photorealistic images of non-existent faces, landscapes, or even artworks. For instance, DeepArt's GAN-based algorithm can transform simple sketches into intricate paintings resembling the styles of famous artists like Picasso or Van Gogh.
In the field of entertainment, GANs are reshaping the way content is created and consumed. For instance, AI-generated music and literature are gaining traction, with algorithms capable of composing symphonies or writing stories that captivate audiences. Additionally, GANs have been employed in video game development to generate realistic environments, characters, and even entire game levels.
Ethical Considerations: While GANs offer tremendous potential, they also raise ethical concerns, particularly regarding the generation of deepfakes—synthetic media that convincingly depict individuals saying or doing things they never did. This has significant implications for misinformation and privacy, underscoring the importance of responsible AI development and regulation.
Future Directions: As research in GANs continues to advance, the possibilities seem boundless. From enhancing creative workflows to aiding scientific discovery, GANs hold the key to unlocking new frontiers in AI. Moreover, with developments in areas like unsupervised learning and reinforcement learning, GANs are poised to become even more versatile and impactful in the years to come.
Generative Adversarial Networks represent a paradigm shift in AI, enabling machines to exhibit creativity and produce content previously exclusive to human creators. As we delve deeper into the realm of GANs, it's crucial to foster a balance between innovation and ethical considerations, ensuring that this transformative technology serves the betterment of society.
In conclusion, Generative Adversarial Networks stand as a testament to AI's boundless potential, offering a glimpse into a future where machines not only assist but actively participate in the creative process. With continued research and responsible deployment, GANs have the power to reshape industries, inspire innovation, and redefine the very nature of creativity in the digital age.